Safe Ways Utilities Boost Customer Service Performance with AI
- Senior_Editor
- Feb 5, 2024
- 5 min read
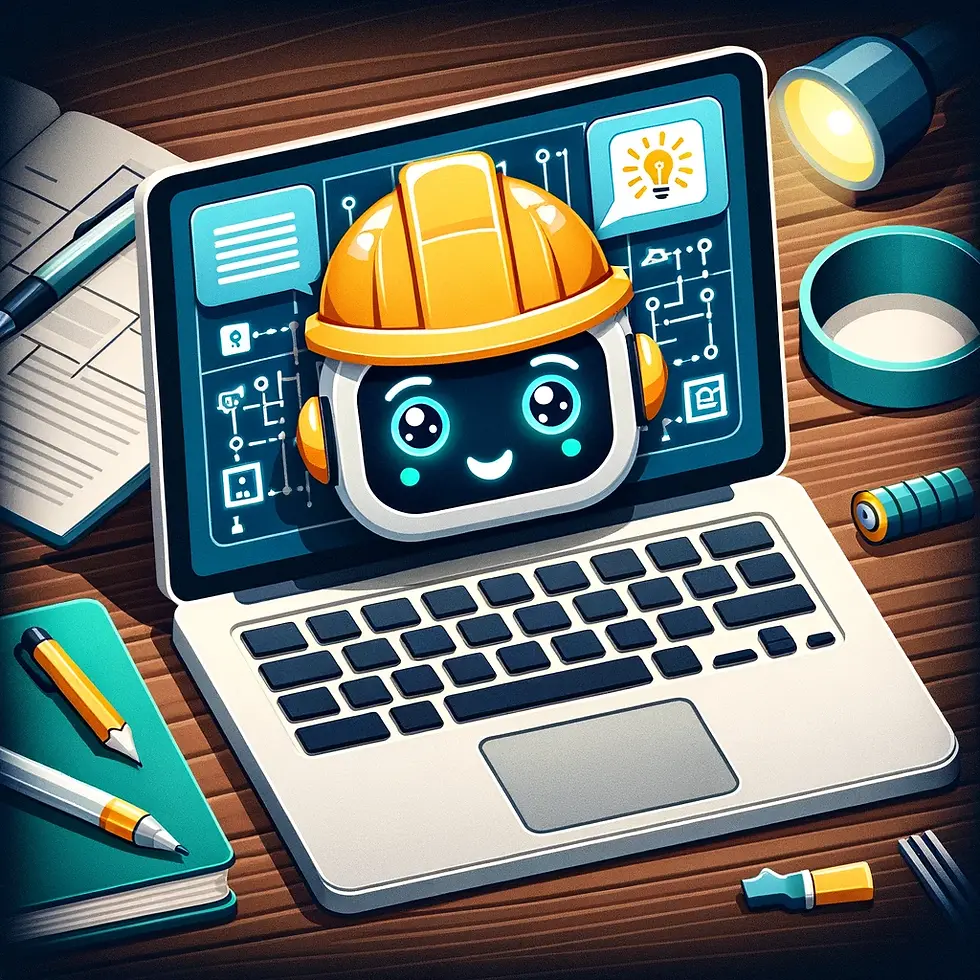
Utilities understandably have taken a “wait and see” approach to implementing AI and chatbots in their customer service centers, especially in customer facing applications. That’s a thoughtful take on this new technology considering that a majority of customers across multiple surveys report being less than thrilled with the typical chatbot experience. Utilities benefit from letting other industries enhance the technology and uncover safe data privacy and business practices while building more mature customer experiences.
Utilities investing in technology will see much better results in customer service performance by focusing on tools that support the needs of their customer service representatives on KPI like first call resolution, reduced average handle time, customer satisfaction, and CSR productivity. Tools that help agents “be right the first time” and deliver more accurate information to customers more consistently across the platform make for sound technology investments.
That’s EXACTLY where AI tools can benefit utility customer service teams today and should be a part of your technology roadmap. Specifically, investments in tools that leverage the analytical and pattern matching capabilities of machine learning and natural language query and response features of large language models support utilities with safe, controlled, and effective technology to dramatically improve the performance of your customer service operations.
All large language models get “trained” on a large volume of text data to understand the relationship between words and phrases used in language. The training data helps the LLM learn context for various conversations with users, and the scale of the training helps the LLM be more accurate in making connections and associations within the data set. In short, by training and LLM with a specific context, it gets smarter about the topic and more able to answer questions that make sense.
So, imagine a utility centric LLM trained on the transcripts of 500,000+ conversations that utilities have had with their customers, redacted to maintain customer privacy. LLMs allow utilities to unlock the storehouse of knowledge currently locked up in the archives of their recorded calls. By transcribing, redacting and training a Large Language model on actual customer service calls, as well as the post-call surveys completed by customers, utilities have an opportunity to create an unrivaled tool for customer service excellence.
Machine Learning and Large Language Models possess capabilities to parse language for patterns and nuanced details at a far greater level than previous generations of technology. These tools detect subtle changes in tone and sentiment, learn patterns and frequency of phrases agents and customers use during conversations, understand the outcome of calls based on language within the call and metadata associated with the call, and predict trends based on interaction of this large trove of useful data.
Once trained, the CX_AI would be able to respond to utility Customer Service professional’s questions on topics that previously depended on broad general analysis or attributes with low confidence such as agent disposition.
For instance, instead of depending on general reports of FCR%, AHT, and Abandonment, utility CS analysts could ask a CX_AI the following questions:
What are the most common topics of the calls to customer service with the shortest duration? What about the calls with the longest duration?
What are the 5 most common topics of calls with severe negative customer sentiment?
What customer phrases are most often associated with calls with negative outcomes?
What are the most common topics of calls resulting in first call resolutions?
What are the least common topics of calls resulting in first call resolutions?
These insights inform CX leaders on the right priorities for further investigations. For example, a training manager would likely follow up this line of queries with more investigation to identify key phrases during long calls with negative outcomes to adjust training for specific coaching to reduce customer tension and improve changes for positive outcomes.
It’s just a matter of adjusting the question a user poses to the CX_AI, such as:
On calls that do not result in first call resolution, what are the most common phrases that customers use that also appear on calls with poor customer sentiment?
Compare the calls where these phrases appear with calls ending with positive outcomes. What phrases do customer service representatives use to improve the outcome of the call?
What phrases do agents use that seem to improve the customer sentiment most effectively during long calls?
Are customer service representatives properly demonstrating empathy on calls when customers express anger or frustration?
What percentage of calls with negative sentiment coincided with CSRs providing a portion of program information that was incorrect or not consistent with utility policy?
Because AI tools can parse language with accuracy at this detail, utility CX teams can more effectively diagnose issues across the entire CS ecosystem instead of relying on limited inspection of small volume of calls.
Operations teams can leverage the predictive capabilities of a CX_AI to investigate trends in calls that may affect service delivery. The predictive analytics features of advanced AI solutions trained with utility data informs Ops teams to take proactive measures to interrupt negative trends, improve outcomes, and promote likely best options for digital self-service. For example:
What are the most common trends in customer service calls each month over the last 12 months?
Based on the trends of the last 12 months and the call outcomes, what types of calls are most likely to result in first call resolutions? What types of calls are least likely to result in first call resolutions?
Of the calls least likely to result in first call resolution, what are the most common complaints in the process according to customers?
How effective are our customer service representatives at resolving complex issues compared to routine inquiries?
How consistent are customer service representatives at resolving routine inquiries across all of our CSR teams? Does consistency of resolution affect customer sentiment or overall customer satisfaction in routine inquiries?
How consistent are customer service representatives at resolving complex issues across all of our CSR teams? Does consistency of resolution affect customer sentiment or overall customer satisfaction in complex inquiries?
AI tools like these give business leaders the ability to ask questions of their data in “plain language” and receive answers immediately that would require a traditional business intelligence approach days or weeks to assemble iteratively. With an AI trained with business data in this fashion, analysis occurs in more conversational fashion leading to broader understanding and faster realization of insights. We see these tools working in coordination with traditional data analysis to inspect and verify the insights, without causing Operations, QA, and Training teams to wait to take steps to inspect and enhance their own business processes.
An AI trained with data from customer calls redacted for privacy and informed with metadata from the telephony systems would deliver an incredible advantage to Customer Service operations teams to enhance the performance of their CSR teams. The current environment of matrix style KPI dashboards and ad-hoc reports provides limited insights to quality, training and operations, so Customer Service teams make limited performance improvements. Imagine what those teams could do if they had a resource to answer their questions with insights informed by thousands of data points from actual customer conversations connected across different dimensions in real time without the difficulty of building complex BI queries. Simply have a conversation with a trained CX_AI about the data to develop “plain language” insights about trends in CS operations that Operators can use to improve performance immediately.
We think AI in this type of application offers utilities the opportunity for safe, effective, highly controllable experiments in technical innovation and performance improvement with direct effect on customer service and satisfaction. The incredible advances in pattern matching, predictive analytics, and natural language processing that AI delivers for users makes it a perfect complement to help Customer Service teams get genuine insights more quickly and efficiently to put to use in the CS Center. It’s worth considering on our technology roadmap.
Image created using DALL-E, a product of OpenAI, created February 5, 2024
コメント